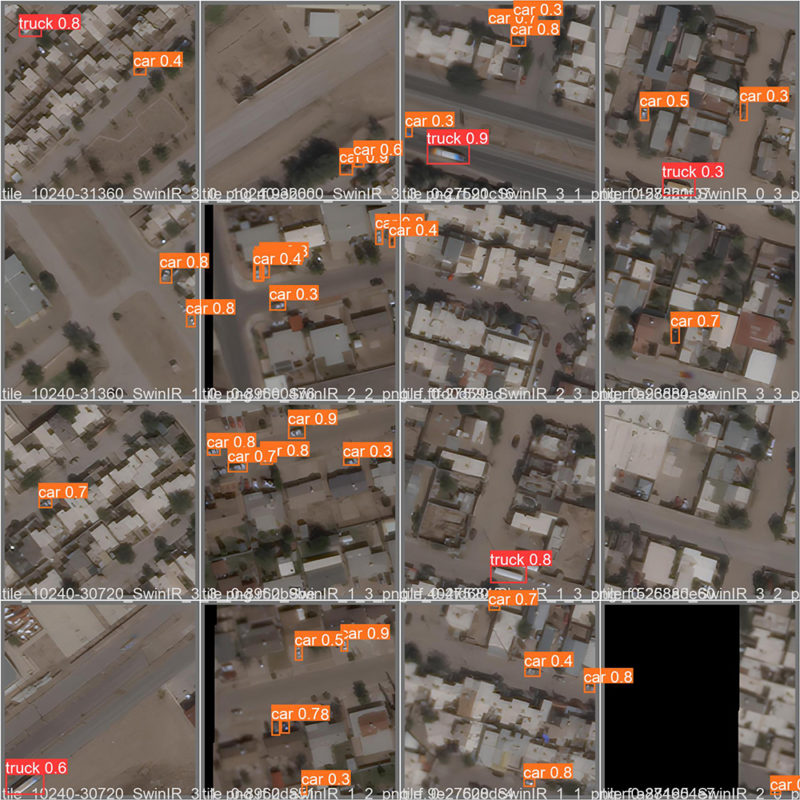
Research recently completed by the Texas A&M Transportation Institute’s (TTI’s) Center for International Intelligent Transportation Research (CIITR) demonstrated the potential usefulness of unmanned aerial systems (UAS) and satellite images, combined with artificial intelligence (AI), for improving accuracy of border travel time estimates in extreme queuing conditions. The land ports of entry (LPOEs) at the U.S.–Mexico border, for instance, often have long wait queues for commercial vehicles, and especially during the most congested periods, wait times are not always reflected as accurately as possible for those waiting in line.
Building on this research, a CIITR team manages the project Aerial Imagery and AI Applications for Cross-Border Transportation Operations. Two of the team members are Valeria Martinez, senior research associate, and Alejandro Berlanga Alzaga, software applications developer I. Martinez and Berlanga work in TTI’s Research & Implementation Program in the El Paso Office and conduct field work as part of this project. Other team members include Rafael Aldrete, principal investigator (PI), CIITR director, and senior research scientist; and Swapnil Samant, co-PI and associate research scientist.
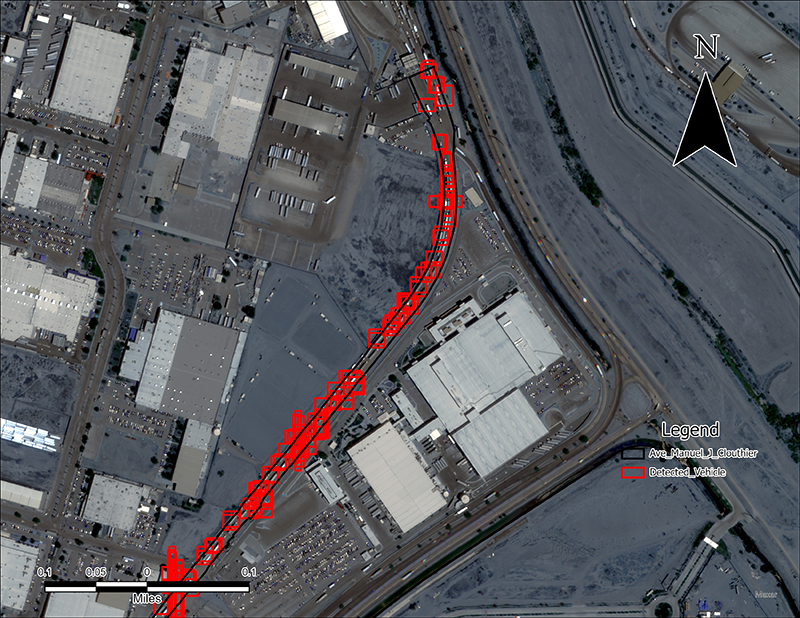
“This project for me is a very exciting one, and I really feel passionate about it,” says Martinez, an unmanned aerial vehicle (UAV) pilot certified by the Federal Aviation Administration. “We have seen these kinds of technology ideas in the past only on TV and in stories, as fictional ideas. Now I get to work on it and live it, having the experience firsthand. And what excites me the most is that this project will only get better in the future.”
The team’s goal is to test and improve methods and AI algorithms from the previous CIITR research and explore other applications for aerial, satellite and AI technologies in transportation operations. Due to recent enhancements in satellite imagery, CIITR researchers can better control the timing and number of images they collect and incorporate additional imaging capabilities. The team is also building 3-D models from the images as another way to present and analyze data.
Researchers conducted a literature review of trends in UAS technology to continue improving images for border-crossing travel time monitoring. They also developed a dataset for UAV and satellite imaging to help machine learning models recognize vehicles in congested spots at LPOEs. The team is assessing other applications for these technologies and methods, as well as identifying challenges of applying UAS and satellite imagery to traffic monitoring and suggesting potential solutions.
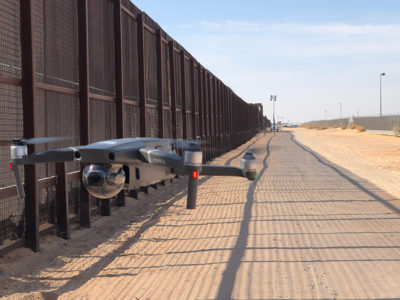
“I love working on this project because I get to work with the latest technologies,” notes Berlanga. “My main role on this project has been as the AI specialist — exploring new AI algorithms, training models with TTI-curated datasets and analyzing results from different algorithms to identify avenues for improvement. And we’re using new AI technology to resolve older problems like the accuracy and reliability of wait times at LPOEs.”
Recent advancements in UAS, satellite and AI technologies have garnered interest from traffic operations staff and stakeholders. With a lower cost and an improved ease of use, these technologies present a viable option for collecting border-crossing travel time information. Accurate wait times at LPOEs can, in turn, help commercial vehicles make more efficient trips across the border.
Aldrete notes, “The findings suggest that aerial imagery advances coupled with AI will allow transportation agencies to cost effectively and accurately estimate real-time travel times in congested, slow-moving corridors, including urban highways, seaport roadways and international LPOEs.”