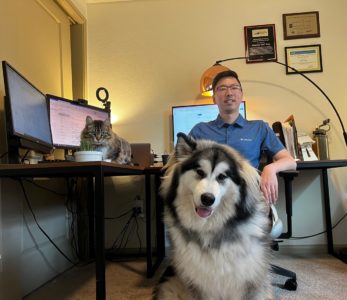
Xiaoqiang “Jack” Kong, Texas A&M Transportation Institute (TTI) graduate research assistant, published the article “Work from Home in the Post-COVID World” in Case Studies on Transport Policy. The transport policy field blends engineering, economics, sociology and law to develop key solutions. Case Studies on Transport Policy pairs theoretical analyses with case studies to better inform decision-makers, planners and practitioners in this field.
Co-authors on the article include Amy Zhang, doctoral student in The University of Texas at Austin’s Department of Sociology; Xiao Xiao, doctoral student in Texas A&M University’s Zachry Department of Civil and Environmental Engineering; Subasish Das, TTI associate research scientist; and Yunlong Zhang, professor in Texas A&M’s Zachry Department of Civil and Environmental Engineering.
Kong notes, “COVID-19 has profoundly changed our society, and its ripple effects have impacted every aspect of our daily lives, including how we work and commute. There is a dire need to thoroughly understand the patterns of adopting the work-from-home behavior in the post-COVID world and its potential impact on commute traffic patterns.”
The article discusses public attitudes about working from home due to the pandemic. The findings reflect various factors and influences, such as a smoother transition to work from home every day for those who had previous work-from-home experience. Policymakers and city planners could use the study’s results to understand and address the public’s opinions about working from home.
“Kong has been working in TTI’s Mobility Analysis Division, and he’s instrumental in several Big Data projects,” says Das. “He’s one of our best Ph.D. students! Since 2020, he has published 20 articles in top-tier journals including Accident Analysis & Prevention, Journal of Safety Research, IEEE Transactions on Intelligent Transportation Systems, Case Studies on Transport Policy, and Transportation Research Part F: Traffic Psychology and Behaviour. In this study, he applied an innovative, unsupervised learning algorithm to identify public perception on ‘work from home’ from well-designed survey data.”